3 lessons to learn before creating your own data team
Dear reader,
Welcome to Christelle’s world, one that’ll likely feel familiar. In this world, 5 years ago, she had a mission to build both the data team and its stack!
A little bit of context
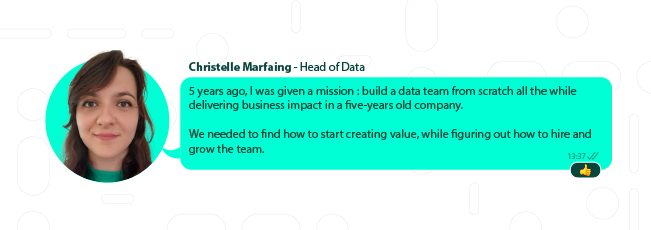
Because of the scarce resources allocated to this topic at the time, most decisions were made relying on intuition.
Today, it’s still hard to find dependable information on the topic, not tainted by any economic agenda of the authors.
Try this: google “should we centralize or decentralize our data teams?” We had to scroll down to the 7th link to find an article not penned by a SaaS company. Clicking that link, we realized the author was none other than the CEO of a major Knowledge Management vendor. The first independent article showed only on the second page of results. That’s also the case for the broader question of: “How to build a data team from scratch?”
That’s why we decided with Christelle to take a step back and share the tips and tricks she wished she had known before.
However, we won’t rely solely on our own experiences because we have our own biases.
Shooting for a wider and more balanced perspective, we surveyed the Modern Data Network, a French community of Data leaders and experts, from which we gathered the answers of 52 different companies. These companies are from the French Tech, with total headcount below 1,000 and Data team sizes ranging from 1 to 200 FTEs.
Based on their answers, we drew 3 main lessons to guide Data leaders in their future decisions.
Lesson #1: Teams and stacks choices are strongly coupled.
We all wish that business needs directly determine technical stacks, and the team that comes with them. This way, alignment throughout would be perfect. Reality is often quite different, with legacy to handle and additional constraints to deal with.
Data leaders must be aware of the strong coupling between how they build their team, and the underlying stack these teams operate.
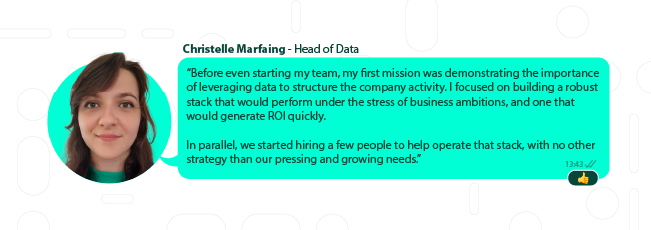
That was the first “aha” moment for Christelle:
There is a strong coupling between the organization of a team — from the picking of profiles, to the design of its processes — and the choice of the technical stack it can operate.
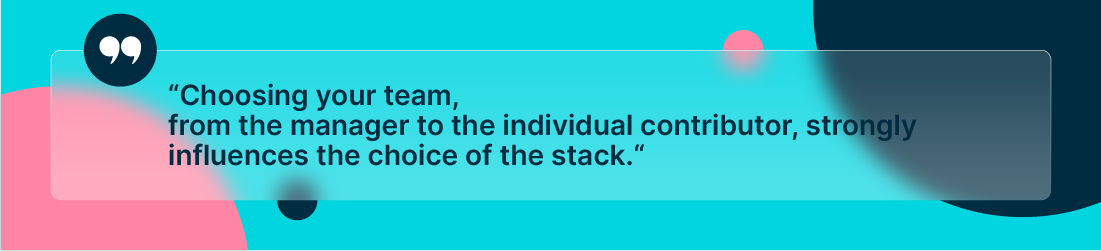
We illustrate this coupling by focusing on the link between building a team and “Make or Buy” decisions.
The Make or Buy decisions hinge strongly on the profile of the Data team leader.
Let’s go back to our survey. Among the respondents, 57% are headed by engineering profiles. This strongly impacts the likelihood to build internal tools over buying off-the-shelf ones.
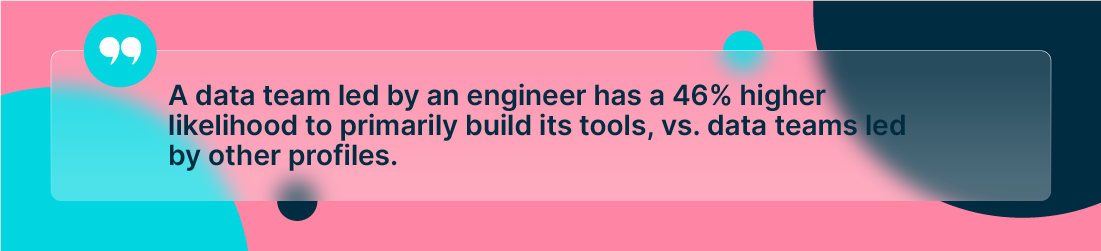
The more you buy, the less you hire… Or not!
A typical argument in favor of buying tools is that it reduces hiring needs. If we follow this Ariane’s thread, that would mean that a team managed by a business profile tends to have lower headcounts.. Reality is more complex.
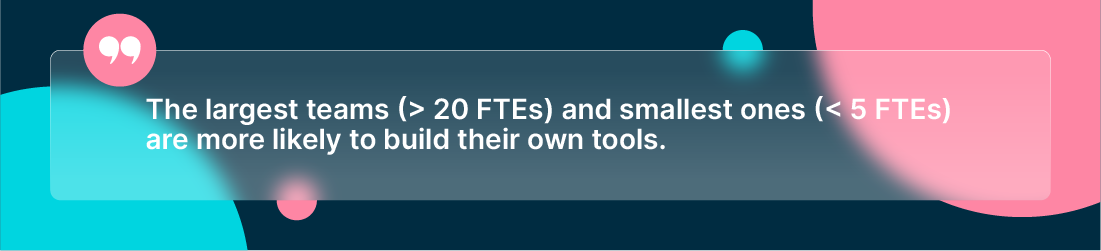
One explanation is that small teams lack the budget to contract costly SaaS solutions, or the bandwidth to manage many of them. Largest teams have the required staffing to build high-quality, tailored solutions with lower costs than off-the-shelf kit.
Adopting a “Make” strategy makes decentralizing a data team harder.
Indeed, maintaining a suite of internal tools is an uphill battle. The team must provide a good UX to internal customers in business teams spread throughout the company, while keeping costs low. This is tough if you don’t have a plethoric central team to support the decentralization while keeping internal tools.
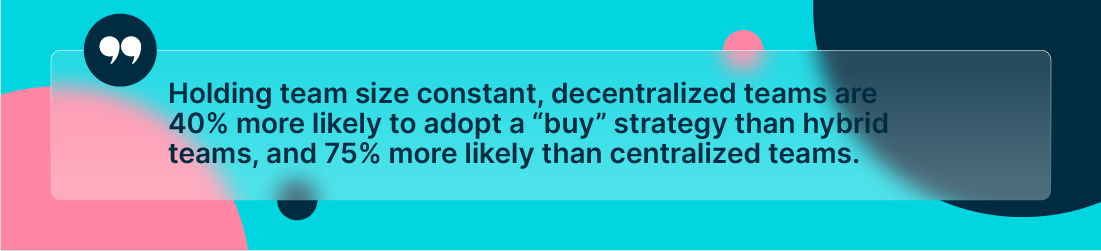
Decentralizing might thus increase IT costs, arising from a growing need for SaaS licenses.
All these elements have to be factored in when picking a Make or Buy strategy, as they impact the team as well.
Lesson #2: reorganization costs are mostly human capital.
In the 2010s, having a data team was an odd idea in companies whose core product wasn’t data-driven.
When data became a priority, centralizing resources became the go-to strategy to build proficient teams, capable of delivering projects and impact quickly.
Today, there is a shift towards decentralization. But it’s no longer a synonym for siloization. Decentralization creates smaller, independent, multidisciplinary teams, with a central unit in charge of coordinating common processes and practices among teams. That’s the data mesh paradigm.
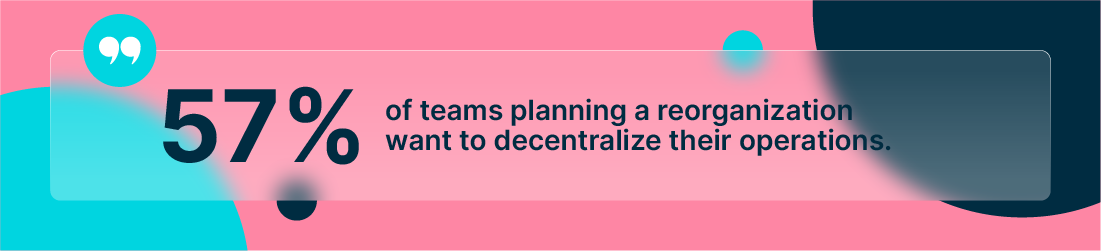
NB: if you want to learn more on this topic, here’s an article on BlaBlaCar’s implementation of their data mesh.
Does this mean you should hurry up to follow the crowd, achieving maturity and productivity gains? Hold on!
Never underestimate the human cost of a reorganization.
To be clear, reorganization is a natural process constantly happening in companies.
And yet, reorganizing is taking non-negligible HR risks. And provided the coupling between the stack and the team, reorganizations also present technical challenges.
It’s thus important to ensure the target organization matches the company’s needs and the historical dynamic of the data team. In other words: a reorganization must answer a clearly defined problem.
Hybrid model vs. decentralization?
Starting at 15 FTEs, two organization paradigms are possible: a fully decentralized one and a hybrid model.
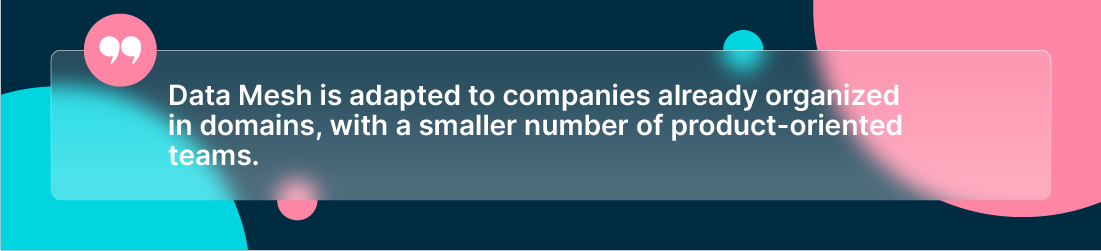
It’s very hard to shift multidisciplinary teams into each domain when these domains are poorly defined. For instance if there are many different internal clients with a low level of data maturity. The risk is to end up with bloated teams, and fall back to the decentralization problems from the 2010s.
In the end, opting for a decentralized model requires a strong knowledge management culture, as well as deep connections between teams to fight against siloization.
Lesson #3: Growing a team is much more than hiring.
Building a team is first and foremost a HR exercise.
Here’s Christelle’s feedback on this:
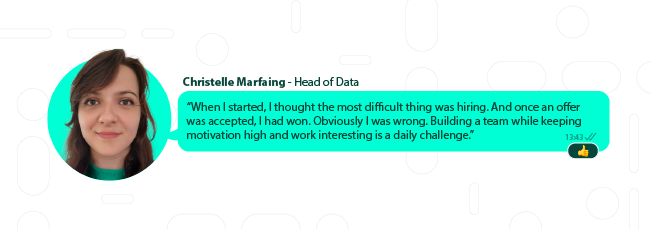
This challenge is transverse to all domains, but strongest when competition for talent and technical complexity are as high as in the data industry.
Moreover, in start-ups without strong HR support, managers have to devote a lot of time to building career ladders, especially as they hire fresh graduates.
One of the first challenges managers face when they grow their teams is specialization. A few figures from our survey to illustrate this point:
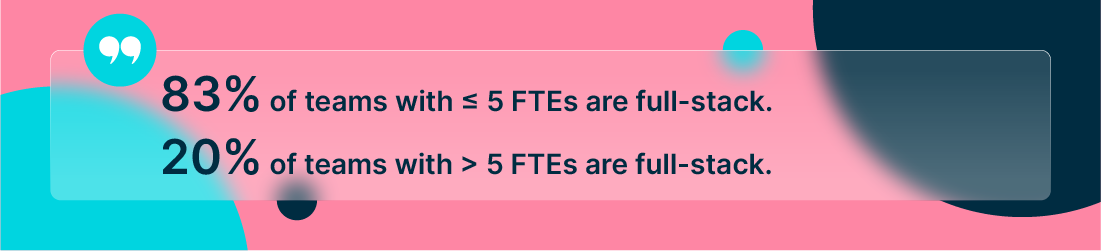
The way from generalist to specialist goes through multiple paths, from re-hiring the whole team to intensive training of existing members on specific expertise areas.
To achieve the switch, the manager has to clearly explain to their teams what the company’s expectations are. There are two major ways.
Have clear, measurable objectives.
These objectives can target projects in the product roadmap as well as personal development exercises.
Simple stuff, but it gives a direction to follow and a source for motivation.
It includes team members in something larger, giving purpose to their work while being explicit on how to grow.
It was a clear output from the survey:
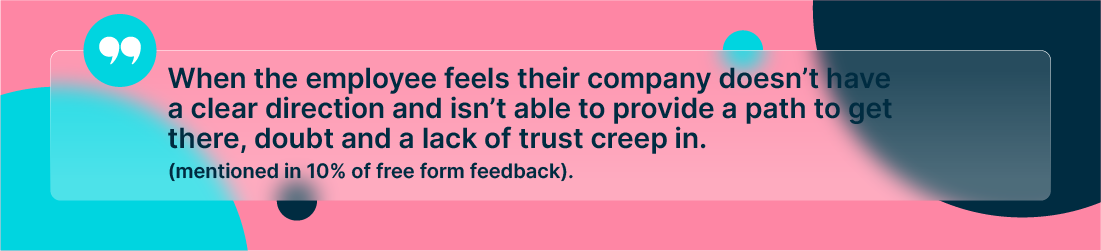
Implementing a clear framework for expected competencies at each seniority level.
The infamous competency matrix. Though efficient, it can be a double-edged sword. To wield with care!
First, what goes inside the matrix must be explicit. Otherwise, the grid creates frustration; it is interpreted as managers’ way to dodge promotions, hiding behind the formalism of the matrix.
Second, you must abide by the rules. If the grid shows someone deserves a promotion and it doesn’t happen, this increases churn.
Third, building the appropriate competency grid is difficult. It’s important to include both soft and hard skills. For instance, growing communication and mentoring abilities is capital for senior profiles: they are expected to be technical experts, and coaches for newcomers.
Last, it’s important to separate and assess both the achievement of objectives and how they are delivered. This highlights compromises between delivery speed and quality; managing this compromise well is the target. Recognizing this balance avoids creating the wrong incentives to prioritize speed over quality.
To conclude, the competency framework is a lever to grow your team for the challenges they face. It’s a key element highlighted by Google in their Project Oxygen; this project enumerates the key success factors of top performing managers. The 6th one is “Supports career development and discusses performance”.
It’s all the more important when we reflect upon the rationale behind reorganizations:
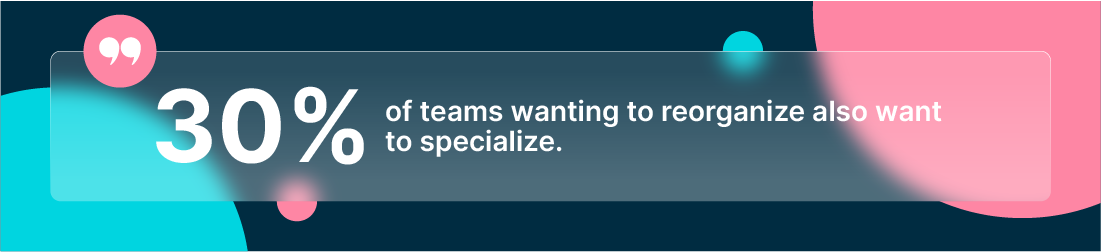
Conclusion: data is made by people
Our takeaway from these three learnings is how critical human factors are in the daily challenges of a data leader.
Fresh out of an engineering school, managers are biased towards seeing every challenge as a technical problem in want of a technical solution.
Reality is different. Even technical solutions are strongly determined by the human factor of the team profiles.
There is a strong coupling between the human capital of a team and the technical or organizational choices managers make.
Understanding this early on in building a team and its stack will help you avoid some of the commonest pitfalls.
Over to you, and good luck!
Cela pourrait vous interresser
Partageons ensemble notre savoir
Nos prochains événements
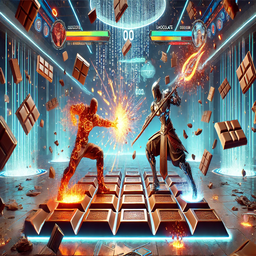
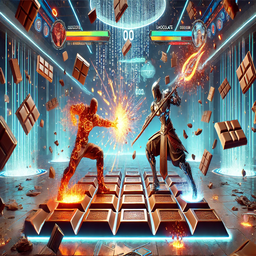
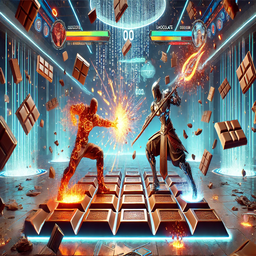